Detecting roads from space
One of the human pressures on biodiversity are roads. In the GLOBIO model (global biodiversity model for policy support), the information on the location of roads is taken from the Global Roads Inventory Project (GRIP) data set. Although GRIP provided a significant improvement over existing global road data sets (Meijer et al., 2018), the data set is still spatially and temporally incomplete. The aim of this pilot study was to test the potential of automatic road network data extraction from remote sensing imagery to create a more complete and up-to-data global road network data set. This report describes the results from the pilot study, discusses model performance and identifies opportunities for improving automatic road network data extraction for global applications.
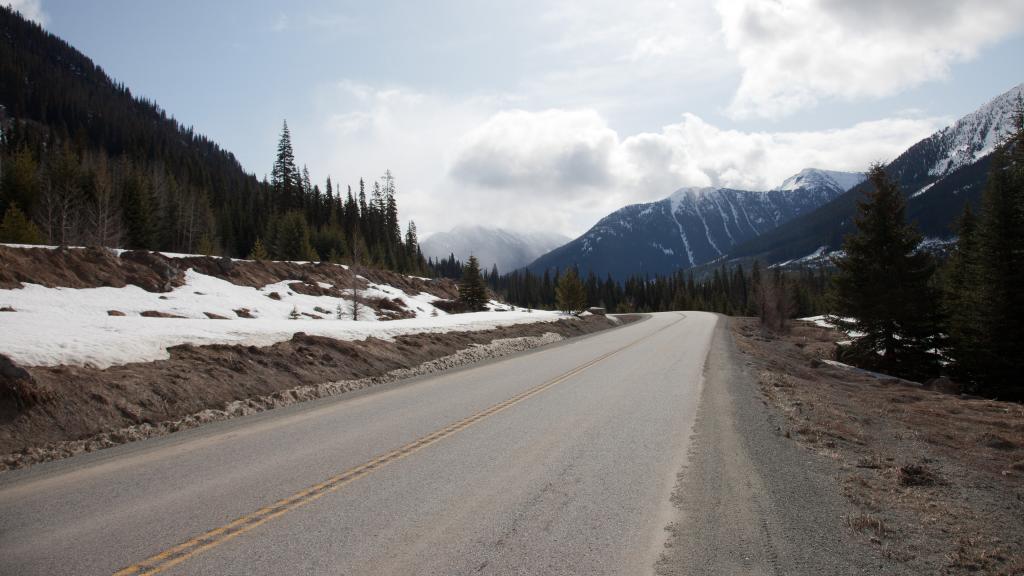
Automatic road network data extraction from remote sensing
The aim of this study was to test the potential of automatic road network data extraction from remote sensing imagery to create a more complete and up-to-data global road network data set. To that end, a recently published deep-learning model (Stewart et al., 2020) was applied to globally available Sentinel-1 SAR imagery. The model was tested based on two study regions with differing road networks and environmental conditions: Division No. 14 in Alberta, Canada, and the Sangha Department in the Republic of Congo. Existing publicly available road data were used for model training. Various Sentinel-1 SAR polarisations were tested as input to the model, namely vertically emitted and vertically received radiation (VV), vertically emitted and horizontally received radiation (VH), and a combination of the two (VV & VH).
Method and accuracy
With VH as input, the model showed the highest completeness (65% and 53% in Canada and Congo, respectively) and overall accuracy (F1=0.48 and 0.44, IoU=0.65 and 0.61). Not all roads in an area were detected by the model (false negatives) and non-roads were occasionally classified as roads (false positives). Overall, it was easier for the model to detect large primary roads than small local roads. The causes of false positives and false negatives are similar to those mentioned in other studies on road detection. False positives were mainly caused by objects with reflectance and spatial characteristics that are similar to those of roads (e.g. grass strips, rivers, terrain edges), while false negatives were mainly due to complex surroundings (e.g. roads and surrounding fields having similar values on the SAR image) or vegetation co. Compared to results from the GRIP project, our model results were positionally more accurate, complete and up to date. However, they do not distinguish between road types.
The method tested here is a prototype and further improvements are needed to increase the accuracy. Improvements may include the use of optical Sentinel-2 imagery or more extensive post-processing (connecting road segments and removing false positives). Pending further improvements in accuracy, the methodology presented here has the potential to provide a robust, cost-effective, and scalable approach to the mapping and monitoring of road networks.
Authors
Specifications
- Publication title
- Detecting roads from space
- Publication subtitle
- Testing the potential of Sentinel-1 SAR imagery and deep learning for (global) road mapping
- Publication date
- 10 March 2022
- Publication type
- Report
- Page count
- 47
- Publication language
- English
- Product number
- 4417